Dr. Tobias Baur
Phone: | +49 821 – 598 2305 |
Email: | tobias.baur@uni-auni-a.de () |
Room: | 2044 (N) |
Open hours: | By agreement |
Address: | Universitätsstraße 6a, 86159 Augsburg |
Links
Research Topics
- Affective Computing
- Artificial Emotional Intelligence
- eXplainable AI
- Semi-Supervised Active Machine Learning
- HCI
Current Lectures
name | semester | type |
---|---|---|
Interactive Machine Learning for Healthcare Lab | winter semester 2024/25 | Vorlesung |
Awards
- 8th International Conference on Affective Computing & Intelligent Interaction (ACII 2019) - Best Paper Award
- ACM Multimedia 2013 Open Source Challenge - Honorable Mention
- UMAP 2013 - Most Participative Demo Award
- IDGEI 2013, FDG - Best Paper Award
Talks / Keynotes
Keynote/Tutorial
"eXplainable Cooperative Machine Learning - An interactive Human/Machine Annotation Process"
International Workshop on Corpora And Tools For Social skills annotation 18.Oct.21 (CATS2021)
23rd International Conference on Multimodal Interaction ( ICMI 2021)
"NOVA - A tool for eXplainable Cooperative Machine Learning"
ECML/PKDD Summer School Würzburg ( EPSS19)
Demonstration
"I see what you did there: Understanding when to trust a ML model with NOVA"
8th International Conference on Affective Computing & Intelligent Interaction ( ACII 2019)
Tutorial
"Social Signal Interpretation for Virtual Agents: Basic Concepts and Implementation in the SSI Framework"
Fifteenth International Conference on Intelligent Virtual Agents ( IVA 2015) Delft, Netherlands, 2015
Networks / Memberships
- Association for the advancement of Affecitve Computing ( AAAC)
- IHK AI Network für die bayerisch-schwäbischen KI-Verantwortlichen ( AI-Network Augsburg)
PC Member / Editorials
- Programme Committee member: AAMAS-2021: 20th International Conference on Autonomous Agents and Multiagent Systems
- Guest Editor: Multimodal Technologies and Interaction: Special Issue "Modeling Interaction with Virtual Characters"
- Programme Committee member: MRC 2020: Eleventh International Workshop Modelling and Reasoning in Context, Santiago de Compostela, Spain, June 8-12, 2020
Software Projects
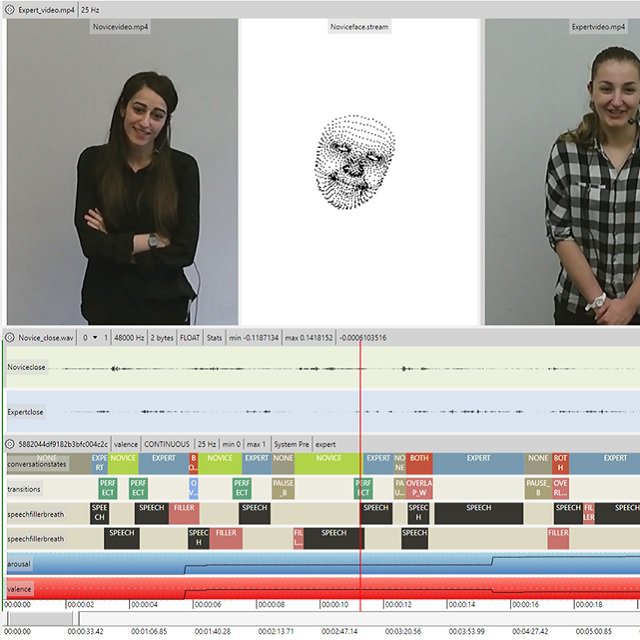
NOVA
NOVA is a tool for annotating and analyzing behaviours in social interactions. A main feature of NOVA is that it allows to employ a collaborative annotation database where annotation work can be split between multiple sides, but also between a human annotator and a machine by supporting human annotators with machine learning techniques already during the annotation task - A process we call Collaborative Machine Learning.
NOVA allows framewise labeling for a precise coding experience, and value-continuous annotations for labeling e.g emotions or social attitudes. The interface is customizable and allows loading and labeling data of multiple persons. The Cooperative Machine Learning capabilities allow to train and evaluate machine learning models, such as Support Vector machines or Artificial neural networks directly from the interface with both, a session completion step, where a model is trained on the first minutes of an annotated sessions to predict the remaining session, and a session transfer step where a model is trained on multiple sessions to predict completly unknown data. With the help of human input the models can then be refined. NOVA further integrates eXplainable AI algorithms to give inisghts into a Machine Learning model’s inner workings.
Article on eXplainable Cooperative Machine Learning with NOVA at KI-Künstliche Intelligenz
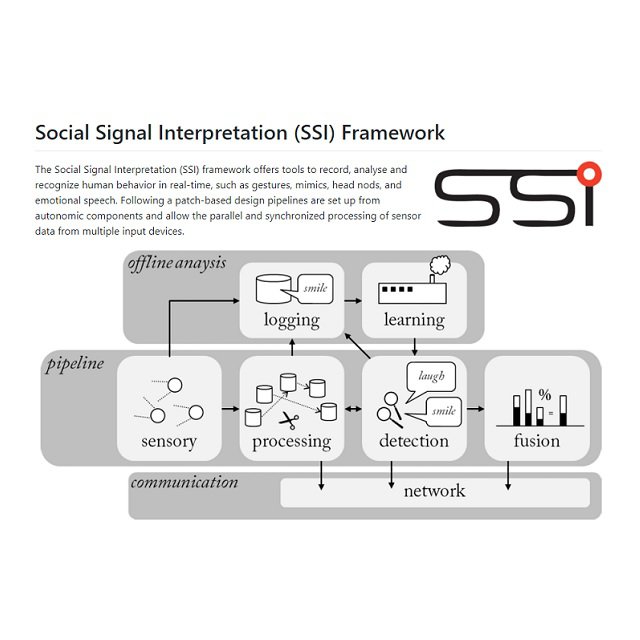
The Social Signal Interpretation Framework
The Social Signal Interpretation (SSI) framework offers tools to record, analyse and recognize human behavior in real-time, such as gestures, mimics, head nods, and emotional speech. Following a patch-based design pipelines are set up from autonomic components and allow the parallel and synchronized processing of sensor data from multiple input devices. In particularly SSI supports the machine learning pipeline in its full length and offers a graphical interface that assists a user to collect own training corpora and obtain personalized models. In addition to a large set of built-in components SSI also encourages developers to extend available tools with new functions. For inexperienced users an easy-to-use XML editor is available to draft and run pipelines without special programming skills. SSI is written in C++ and optimized to run on computer systems with multiple CPUs.