General contact details
Address:
Chair of IT Infrastructure for Translational Medical Research
Faculty of Applied Computer Science
University of Augsburg
Alter Postweg 101
86159 Augsburg
Secretariat:
Phone: +49 821 598 -3738
Fax: +49 821 598 -3739
E-mail: sekretariat-misit@informatik.uni-augsburg.de
Building:
Office Centre Messe (BCM) - 9th floor
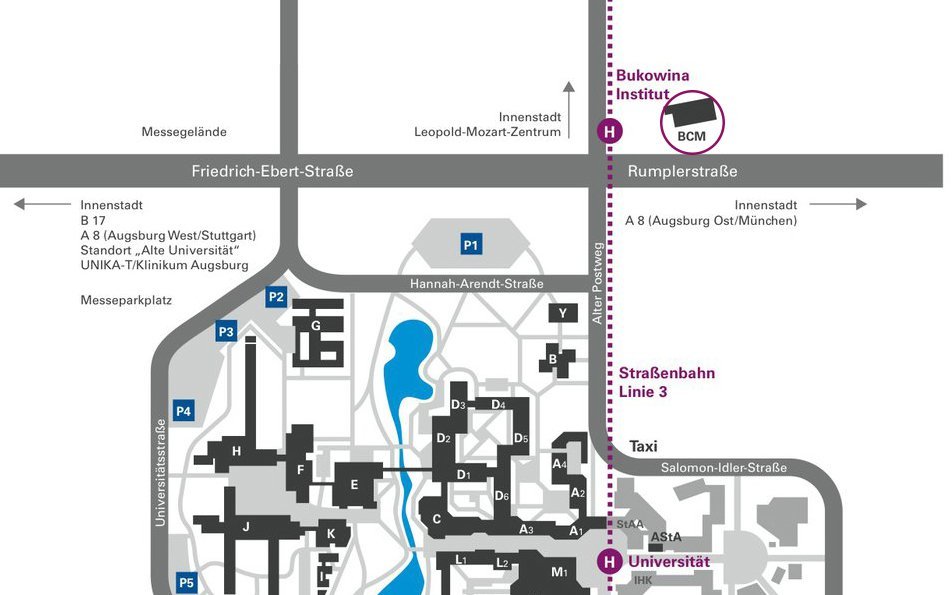